王刚,张瑞昊,刘学龙,袁海东,韩旭.融合稀疏八叉树与卷积神经网络的汽车风阻系数预测[J].计算力学学报,2024,41(1):58~65 |
| 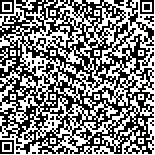 码上扫一扫! |
融合稀疏八叉树与卷积神经网络的汽车风阻系数预测 |
Automobile drag coefficient prediction combining sparse octree and convolutional neural network |
投稿时间:2023-07-30 修订日期:2023-10-23 |
DOI:10.7511/jslx20230730001 |
中文关键词: 风阻系数 深度学习 稀疏八叉树 卷积神经网络 汽车 |
英文关键词:drag coefficient deep learning sparse octree convolutional neural network automobile |
基金项目:国家自然科学基金(12072099;11832011);科技创新2030—"新一代人工智能"重大项目(2021ZD0113100)资助. |
|
摘要点击次数: 413 |
全文下载次数: 276 |
中文摘要: |
针对汽车风阻系数预测研究中参数化方法难以准确表征汽车外造型的难题,提出融合稀疏八叉树与卷积神经网络的汽车风阻系数预测方法。将汽车外造型按照八叉树结构离散,使用平均法向量对离散的复杂曲面进行简化,利用卷积神经网络对八叉树形式的汽车外造型进行特征提取,进而对汽车风阻系数进行快速预测。通过改变卷积层数与全连接层数,研究了不同卷积神经网络结构对风阻系数预测精度的影响。与参数化方法相比,本文提出的外造型表示方法能更好地描述模型细节,构建的卷积神经网络结构对风阻系数预测的最小相对误差为1.453%,且计算速度是CFD仿真的1620倍,具有较高的精度及计算效率。 |
英文摘要: |
This work presents a novel automobile drag coefficient prediction method by combining sparse octree and convolutional neural network (CNN), aiming at the problem that it is difficult for parametric methods to accurately represent the vehicle exterior styling.Based on the octree discrete method, the vehicle exterior shape is first discretized and simplified by using the normal vectors.With the aid of CNN, the exterior styling features are then extracted, which improves the speed of prediction for automobile drag coefficient significantly.By changing the number of convolutional layers and fully connected layers, the influence of different convolutional neural network structures on the prediction accuracy of automobile drag coefficient is investigated in detail.Numerical studies demonstrate that the present method can give more accurate detailed descriptions for vehicle styling compared with the traditional parametric methods.Therefore, it effectively improves the prediction accuracy and calculation efficiency, as the minimum prediction error yielded by the present CNNs is 1.453% and the calculation speed is 1620 times higher than CFD simulation. |
查看全文 查看/发表评论 下载PDF阅读器 |