叶红玲,李继承,魏南,隋允康.基于深度学习的跨分辨率结构拓扑优化设计方法[J].计算力学学报,2021,38(4):430~436 |
| 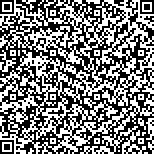 码上扫一扫! |
基于深度学习的跨分辨率结构拓扑优化设计方法 |
Cross-resolution acceleration design for structural topology optimization based on deep learning |
投稿时间:2021-05-09 修订日期:2021-05-26 |
DOI:10.7511/jslx20210509403 |
中文关键词: 拓扑优化 ICM方法 深度学习 跨分辨率加速设计 |
英文关键词:topology optimization ICM method deep learning cross-resolution acceleration design |
基金项目:国家自然科学基金(11872080);北京市自然科学基金(3192005)资助项目. |
|
摘要点击次数: 1546 |
全文下载次数: 466 |
中文摘要: |
在传统拓扑优化设计中,随着结构单元增加,迭代计算过程消耗了大量的时间。本文提出了一种基于深度学习的方法来加速拓扑优化设计过程,缩短了结构拓扑优化设计的迭代过程,并生成了高分辨率拓扑优化结构。利用深度学习方法,在低分辨率中间构型与高分辨率拓扑构型之间创建高维映射关系,利用独立、连续和映射(ICM)方法建立深度学习网络所需要的数据集,训练神经网络以实现加速过程,将结构拓扑优化设计问题转化为图像处理中的风格迁移问题。通过引入条件生成对抗式神经网络CGAN(Conditional Generative and Adversarial Network)解决了跨分辨率拓扑优化问题,实验验证了优化过程效率的提高,该方法具有良好的泛化性能,研究模型在其他结构优化设计中具有可推广性。 |
英文摘要: |
In the traditional topology optimization design, iterative calculations cost too much time with the increase of structural elements. In this paper, a cross-resolution acceleration method based on deep learning is proposed to shorten the iterative process of topology optimization design, and to generate a high-resolution topological configuration. A deep learning model is introduced to create a high-dimensional mapping relationship between the low-resolution intermediate configuration and the high-resolution topological configuration, and the dataset is established by Independent Continuous Mapping (ICM) method to train the deep learning model. The topology optimization design problem is transformed into the problem of style transfer in image processing when the pre-trained deep learning model is acquired. Conditional generative and adversarial neural network (CGAN) is used to solve the problem of cross-resolution topology optimization Numerical experiment verifies the feasibility of the cross-resolution acceleration method for topology optimization. The method has good generalization performance, and the deep learning model is generalizable to other optimization design problems. |
查看全文 查看/发表评论 下载PDF阅读器 |